Machine Learning
Nicolas Raoul
November 10, 2018
# What is a neuron? Biological cell that: 1. Takes a few eletrical inputs with coefficients, 2. And based on these, generates an electrical output.
# Neuron 
# Learning - When the result is correct, the coefficients get a bit higher. - When the result is wrong, the coefficients get a bit lower.
# Neural network - A neural network is a group of neurons, with various connections between them. - Some of the neurons are connected to inputs (for instance the eyes or the tongue). - Some of the neurons are connected to oututs (for instance muscles).
# Neural network 
# Learning 
# On a computer - It is easy to simulate a neuron, or a neural network, on a computer. - Many open source libraries are available. - Now, let's see how to use a library to perform machine learning.
# Goal - Given an input, the goal is to generate the right output, based on a finite sample of input/output couples. - Examples: - Based on the content of a document, tag it as "work" or "private". - Based on a website visitor navigation, recommend products they are likely to want.
# Steps 1. Gather a lot of sample data, ideally several thousand samples, more for difficult concepts with many inputs or many outputs. 2. Choose a machine learning library that fits your needs. 3. Train it using 80% (typically) of the sample data. 4. Validate/test it using 20% of the sample data. 5. Use the resulting function (obscure but fast calculation) in production.
# Tools - Numpy: Python numerical library, used for its arrays - TensorFlow: Neural network library, very flexible - Keras: High-level interface over TensorFlow
# What is a tensor? - A **tensor** is a multi-dimensional array. - Example: [[[1, 1, 1], [2, 2, 2]], [[3, 3, 3], [4, 4, 4]]] - The **shape** of a tensor a representation of its dimensions. - Example: The shape of the tensor above is [2, 2, 3] (yes, also a tensor). - Shapes sometimes have unknowns, for instance [[?,100,100,1]. - Tensors are used everywhere in TensorFlow for very different things.
# Neural networks - TensorFlow simulates a neural network: - Inputs - Intermediary layers of neurons - Outputs - All data coming in and out, and through each layer, is tensors.
# Designing a neural network 1. Determine what types of output you want. 2. Make choices after looking at successful implementations for similar problems. 3. Train, validate. 4. Depending on accuracy found during validation, rethink choices or fine-tune, and repeat. 5. Test.
# Design choices - Input resolution, transformations (eg. grayscale) - Number and type (dense/pooling/dropout, conv2D, LSTM, etc) of intermediary layers - Number of neurons in each intermediary layer - Propagation (activation) function: ReLU, softmax, etc - Optimizer algorithm: GradientDescent, Adam, etc - Number of training epochs
# Ex: VGG16 2014 
# Ex: Xception 2016 
# Hyperparameters - Finding the right hyperparameters (design choices) takes time, experience is important. - Some tools can help speed up the search for the optimal hyperparameters: https://github.com/hyperopt/hyperopt
# Practice - Go to https://playground.tensorflow.org - Run simulations. - Play with the parameters. - Try to solve problems using as few neurons as possible. - Make your neural network resistant to noise.
# Hands on
# Getting started 1. sudo apt-get install virtualenv 2. virtualenv tensorflow_env 3. pip install tensorflow keras 4. Write and run Python script, for instance https://www.tensorflow.org/tutorials/keras/basic_classification
Selfie:  Not selfie:  
# Getting data 1. Downloaded sample pictures from Wikimedia selfie categories. 2. Downloaded random pictures. 3. Manually fixed misclassified pictures. 4. In total I gathered 291 selfies and 1102 non-selfies. 5. More data would lead to better prediction.
# Splitting the data - 80% for training, 10% for validation, 10% for test. - If you have millions of samples, using most of the data for training is acceptable. - Never use validation pictures for training, or test pictures for validation, or vice versa.
# Design choices - Convert images to grayscale. - Resize all images to 20 pixels x 20 pixels. - Neural network with 4 layers.
# Code 1. Loading and pre-processing of the data. 2. Definition of the model (see next slide). 3. Training. 4. Validation. 5. Output weights (to use in production) and statistics.
# Code ``` model = keras.Sequential([ Conv2D(filters=64, kernel_size=2, padding='same', activation='relu', input_shape=(thumbnail_height, thumbnail_width, 1)), MaxPooling2D(pool_size=2), Dropout(0.3), Conv2D(filters=32, kernel_size=2, padding='same', activation='relu'), MaxPooling2D(pool_size=2), Dropout(0.3), Flatten(), Dense(256, activation=tf.nn.relu), Dropout(0.5), Dense(1, activation=tf.nn.sigmoid) ]) model.compile(optimizer=tf.train.AdamOptimizer(), loss='binary_crossentropy', metrics=['accuracy']) ```
# Code - Full source code at https://github.com/nicolas-raoul/selfie-or-not - Running for 10 epochs takes 1 hour.
# Results Accuracy on test data: 86%

# Actually using ML
# Deploying to production 1. Export from Python: `tf.saved_model.builder.SavedModelBuilder` 2. Import from Java: `SavedModelBundle.load` Also usable from other languages such as JavaScript.
# Performance (training) - Very slow, very CPU/GPU-intensive, memory-intensive if using a big network or big batches - Tips: Use a GPU, compile TensorFlow to match your hardware
# Performance (production) - Extremely fast - Low memory - Size in Java is 80 MB, but can be reduced to 1.5MB
# When to use ML? - When implementing a function: - with few inputs and few outputs, - that does not modify any state nor write anything, - with no human interaction nor configuration. - Must have at least 1000 input/output samples for each class.
# When NOT to use ML? - When a performant algorithm could be written in less than a few weeks. - When errors would have harmful consequences.
- ML lacks ethics, unchecked usage may reinforce society inequalities in a vicious circle. 
- Neural networks are opaque, do not use them in situation where transparency is needed. 
# What will you use ML for? 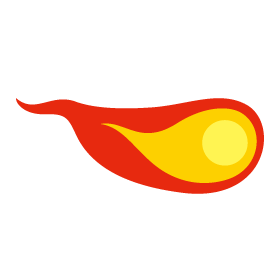